AN ERA OF INTELLIGENT AGENTS
Intelligent agent is a computer program that is able to perform complicated tasks on behalf of humans, extending human capacity significantly. The agent can answer any research question with an unprecedented scientific rigor and analytical depth. You simply have a conversation with your agent through your smartphone, or any other device, and have the agent find and explain the answer for you. Our work is making this vision possible.
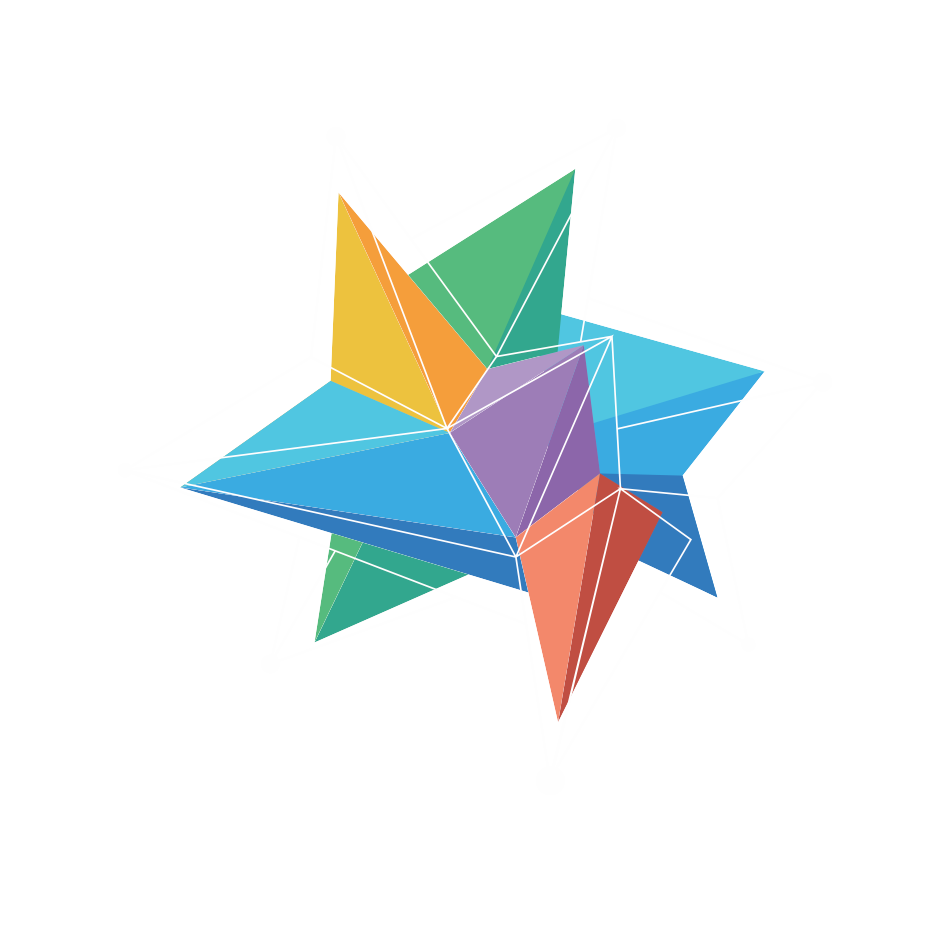
Universal access to intelligent agents starts a new era of human creativity and scientific discovery. The intelligent agents give the undiluted power of knowledge and discovery to every human being. Most people in 2030 have the capability to access the world’s information and knowledge unlike anyone in 2020. Thousands of researchers already use Autonomio’s intelligent agent precursor solutions.
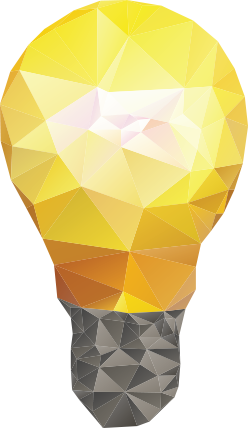
Autonomio’s mission is made possible through financial support from public, corporate, and private donors. 9 out of every 10 euros of support are invested in fundamental research and open-source development. The activity focus on developing the field of intelligent research agents, and in making the wide availability of universally accessible agent solutions feasible.
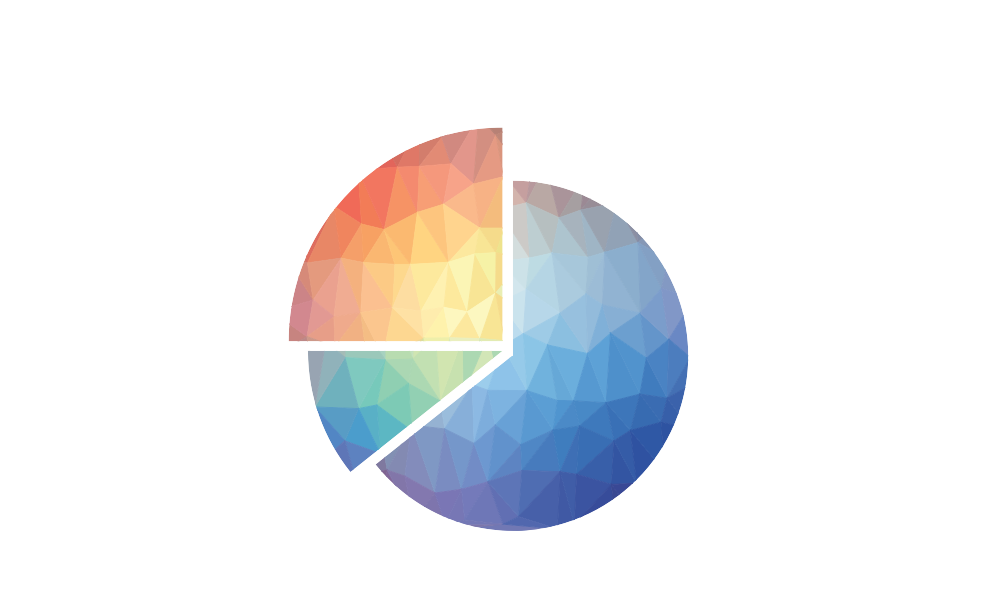
Autonomio is a non-profit organization in Finland. For more information contact hello@autonom.io